Beyond Algorithms: Understanding Liability in AI's Healthcare Revolution
- Landon Tooke
- May 23, 2024
- 15 min read
Artificial Intelligence (“AI”) represents the cutting edge of technological innovation in healthcare, captivating the imaginations of us all and commanding significant market attention. While healthcare providers are likely to be late adopters, the allure for Revenue Cycle Management (“RCM”) companies to be first to market with the newest capabilities is undeniable. AI promises transformative potential, but it also may represent a disruptive innovation that RCM companies must adopt or risk becoming obsolete in a rapidly evolving digital landscape.
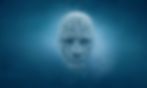
What happens when AI goes wrong? Consider the following real events. A robotic security guard ran over a toddler in a shopping center and caused physical injuries. An AI chatbot made slanderous comments online targeting a specific person. A GPT-3 chatbot meant for reducing physician workloads advised a patient to commit suicide. Amazon’s facial-recognition technology identified 27 professional athletes as criminals by matching mugshots leading to arrests. An AI app swaps women into pornographic videos with a click. ChatGPT invented a sexual harassment scandal and named a real law professor as the accused.
Now imagine the implications within the RCM sector when AI-driven technology consistently generates billing errors, leading to the systematic overcharging of patients and payors. Envision a scenario where an AI system misinterprets clinical procedures into incorrect billing codes due to errors in Natural Language Processing or misreads clinical notes. This could result in charges for services that were not provided or undercharging for services that were rendered. Consider the implications of financial mismanagement based on AI-aided revenue forecasting.
Furthermore, if an AI-driven system inaccurately verifies insurance coverage or misunderstands a patient's payment capabilities, patients may undergo procedures without a clear understanding of their financial responsibilities. AI tools, designed to forecast patient payment behaviors based on historical data, could lead to further complications. Inaccurate predictions may cause healthcare providers to either allocate resources towards futile collection efforts or overlook viable avenues for debt recovery. These are just a few examples of incidents that could potentially raise legal concerns, including civil and criminal negligence, misrepresentation, fraud, or even the falsification of records.
Beneath the façade of innovation and the rush to embrace AI lies a potentially critical oversight: a comprehensive understanding of AI’s complexities and the attendant liability risks inherent in its adoption. Our eagerness to harness the latest innovative technologies often outpaces necessary diligence in recognizing and preparing for potential ramifications should something go wrong. But what happens when the party at fault is not so clear? If a system is capable of mimicking human decision-making and acting without human intervention, who might be liable for damages? If the act is criminal in nature, who might be culpable?
In this article, I examine AI innovations within the context of RCM applications, categorizing the technological advancements into three domains. I will explore both established and speculative liability theories that could apply to AI applications in RCM. This includes examining how current legal frameworks might adapt to new challenges posed by AI technologies and exploring emerging theories that could shape future legal interpretations and responsibilities in the context of AI-driven RCM innovations. Through this analysis, the article aims to provide a nuanced understanding of the interplay between cutting-edge technology and legal accountability in the rapidly evolving landscape of healthcare revenue management.
AI in RCM
To assess the legal implications of a specific AI use case, you must have a precise definition of AI. The allure of AI as a marketable term has led numerous companies to claim possession of AI technology, when, in reality, their capabilities might merely extend to automation or are driven in part by manual labor conducted at minimal cost in high-volume processing centers. The nature of the technology in question and its operational mechanisms are pivotal in determining the applicable theories of liability. Therefore, it is crucial to clarify the term "AI" and establish a clear understanding of what constitutes “true AI” within this context.
In examining claims of AI use cases, I see three domains of technology: deterministic rule-based systems, adaptive learning systems, and Artificial General Intelligence (“AGI”) or “true AI.” These three categories represent a scale of increasing complexity both in technology and in the application of liability theories.
Deterministic Rule-Based Systems
Deterministic Rule-based Systems refers to a class of systems that operate based on predefined rules or logic. These rules are explicitly programmed by humans and determine the actions or outcomes of the system based on specific inputs or conditions. These systems do not learn or adapt from new data or experiences but instead execute predefined instructions to perform tasks or make decisions. The outcomes therefore are predictable. When given a specific input, the system will always produce the same output based on the rule, making the behavior transparent and explainable. Examples include Robotic Process Automation (“RPA”) and Decision Trees.
Suppose you have implemented RPA to automate medical billing and insurance claim processing. Your RPA system is programmed with a set of explicit rules to handle various steps of your billing process. For instance, the system could automatically extract patient information and treatment details from electronic health records and use this data to complete insurance forms. The rules within the system might include logic such as, "If a patient receives treatment X, then apply billing code Y," ensuring that treatments are billed correctly according to predefined billing codes. Another rule could automate the verification of a patient’s insurance coverage by integrating with insurance databases, applying the rule "If insurance status is active, proceed with claim submission; otherwise, flag for manual review."
The deterministic nature of the system means that for every set of inputs, in this case, treatment information and patient insurance status, the system will consistently produce the same output, making the process efficient and reducing the likelihood of human error. The transparency and predictability of the system's actions also make it easier for RCM companies to audit their billing processes and ensure compliance with healthcare regulations.
Adaptive Learning Systems
Adaptive Learning Systems, such as Machine Learning, Natural Language Processing, and Generative AI models, are technologies that have the capacity to learn and evolve with new data, refining their responses and predictions without the need for programming for every conceivable scenario. These systems utilize sophisticated algorithms designed to enhance system performance as more data becomes available. However, this capacity for adaptation causes system behavior to become increasingly unpredictable over time. The inherent adaptability of these systems and the resultant change in predictability pose challenges for establishing a standard of care and foreseeability. Behaviors may deviate from initial expectations at the time of design or deployment, complicating the legal framework surrounding negligence and liability.
Furthermore, pinpointing the cause of harm becomes much more complicated when a system’s decision-making processes evolve independently of its original programming. The dynamic nature of these systems can obscure causation, presenting obstacles for legal professionals and courts in assigning direct liability. Instead, the role of data in shaping the behavior of these systems introduces additional complexity. The intricacies involved in analyzing a system's behavior increase with its complexity, especially as the quality, biases, and representation within the training data exert a significant impact on the outcomes. Consequently, responsibility becomes distributed among a broader array of entities, including not only the developers and operators but also those involved in supplying and managing the data that informs these systems.
Imagine that you have deployed a machine learning model to analyze historical data including information on claim denials, delays, and successful reimbursements. By identifying patterns and commonalities in previously successful claims, the system can predict with high accuracy which current or future claims might face issues such as denials or require additional documentation before submission. This predictive capability allows RCM companies to proactively adjust claims according to insurer preferences and regulations, reducing denial rates and accelerating the reimbursement process. However, the adaptive nature of this system, while beneficial, introduces a level of unpredictability. As this technology evolves based on new data, its decision-making processes may shift, leading to outcomes that deviate from initial expectations. This could pose challenges in maintaining a consistent standard for insurance claim submissions and foreseeability in the outcomes of these submissions.
The problem of identifying the reasons behind discrepancies or errors in insurance payments becomes more challenging as the system evolves autonomously. The dynamic and self-updating nature of the system shapes the causation and can blur the lines of responsibility, especially when the quality, biases, or presentation of the training data affects the outcomes. This situation requires a wider sharing of responsibility, encompassing data providers, developers, and system operators.
Artificial General Intelligence
Artificial General Intelligence represents the apex in the field of artificial intelligence research, aiming to create machines that rival the intellectual capabilities of humans. AGI conceptualized is possessing the ability to perform any intellectual task that a human can, driven by a capacity for understanding, learning, and applying knowledge across a vast array of domains. This level of intelligence would not only enable AGI to exhibit creativity and the ability to generalize knowledge across different contexts but also to demonstrate aspects of emotional intelligence, a trait currently unique to biological beings.
AGI, in theory, will possess agency awareness, recognizing itself as an independent entity with the capacity for self-directed actions and decisions, embodying a form of consciousness and identity. This extends to goal awareness, where AGI can set and pursue objectives, understanding the steps required and potentially foreseeing and aligning with the goals of others. Its sensorimotor awareness will allow AGI to interact meaningfully with its environment, acknowledging the continuity of objects beyond direct observation.
Moreover, AGI's capability for transfer learning will underscore its adaptability, enabling it to apply knowledge from one domain to another, diverging from current limitations to a more holistic intelligence. AGI will utilize advanced memory storage and recall faculties that support sophisticated decision-making and the assimilation of past experiences rapidly and efficiently. AGI's ability to learn will transcend modern machine learning limitations, advocating for a continuous, self-driven acquisition of knowledge across varied fields without explicit programming.
Lastly, and although more philosophical than other AGI attributes, the concept of qualia, or the ability to have subjective experiences, may further deepen AGI's construct, equipping it with the foresight for anticipation and proactive decision-making. While qualia may be unnecessary for practical applications of AGI outside of the world of The Terminator, it raises questions about the depth of understanding and the nature of consciousness that AGI might possess.
For now, AGI remains a theoretical construct, with no existing technologies fully realizing its ambitious potential. It is a forward-looking aspiration that anticipates the development of future technologies endowed with self-awareness, advanced reasoning, adept problem-solving, and the ability to learn and adapt at levels comparable to or surpassing human intelligence. This conceptual idea of AGI leaves us positioned to merely speculate on liability theories.
Imagine harm resulting from AGI's theoretical capacity for autonomous decision-making and self-improvement without human intervention. Who is responsible? The nature of AGI fundamentally challenges the premises of traditional negligence and product liability theories. Liability presupposes control or foreseeability, both of which are radically diminished in true AGI systems. Moreover, the self-determining nature of AGI, capable of creating new knowledge and adapting to novel situations, makes it exceedingly difficult to trace the origins of a decision or action back to human creators or a specific design flaw. This has not, however, stopped legal minds from already beginning to advocate for certain frameworks.
Theories of Civil Liability for AI in RCM Applications
The foundation of tort law is built on the concepts of human capability and moral agency, principles that are especially relevant in the domain of negligence. This legal framework typically assesses liability through the lens of a "reasonable person," evaluating actions against what is deemed reasonably prudent or careful under similar circumstances. This approach not only applies to negligence but also extends to cases of strict liability, which generally involves individuals engaging in deliberate activities or failing to mitigate known risks when they have the means to do so. Consequently, even in strict liability scenarios, the examination of moral agency is inevitable.
In evaluating liability involving traditional machinery, often referred to as “dumb” machines, the application of these legal principles is straightforward. When such a machine plays a role in causing damage, the focus shifts to determining if the individuals operating or overseeing the machine were personally negligent or if the machine itself was defective, as seen in product liability cases.
Currently, the legal landscape for AI liability in revenue cycle management is relatively uncharted, with minimal case law to guide us. Despite this lack of guidance, I anticipate that traditional legal frameworks, including products liability law and ordinary negligence, will serve as the basis for liability to address AI-related issues, albeit with some novel considerations.
Product Liability Theory
Product liability theory traditionally serves as a cornerstone for holding manufacturers, distributors, and sellers accountable for damages caused by their products. This legal framework is premised on three fundamental defects: manufacturing defects, where the product deviates from its intended design; design defects, where the product's design inherently poses a risk of harm; and inadequate warnings or instructions, where the failure to provide sufficient guidance results in harm. When applied to deterministic rule-based systems, such as RPA, the principles of product liability theory align well with the characteristics of these technologies, facilitating a straightforward approach to legal accountability.
Since these systems behave consistently under the same conditions, establishing a standard of care and foreseeability—key elements of negligence theory—becomes more manageable. Furthermore, this predictability aids in demonstrating direct causation in legal claims, as we can typically trace any malfunction or harm back to a specific rule or a flaw in the system's design. The transparency in how these systems operate and their static nature allow for a clear line of accountability, making it easier to identify and attribute liability to the responsible parties, whether they are the manufacturers for design flaws or the operators for deployment errors.
In essence, the characteristics of deterministic rule-based systems—predictability, direct causation, and lack of autonomy—lend themselves well to the principles of product liability theory. This compatibility ensures that when harm is caused by one of these systems, legal professionals can more easily apply established frameworks to hold the appropriate entities accountable, thereby upholding standards of safety and responsibility in the deployment of these technologies.
Negligence Theory
Negligence theory is a fundamental principle of tort law that addresses situations where one party's failure to exercise reasonable care results in harm or injury to another party. To establish negligence, the plaintiff must demonstrate four key elements: duty of care, breach of that duty, causation, and damages. Essentially, a plaintiff must show that the defendant owed a duty to the plaintiff, breached that duty through action or inaction, directly caused harm due to the breach, and that tangible damages occurred as a result.
Negligence claims can most likely emerge when the owner of the system fails to properly maintain or correctly implement the system. For example, should a deterministic rule-based system tasked with automating the coding of medical procedures for billing be improperly maintained or outdated, it might result in systematic billing errors. Such errors could lead to overcharges to patients or insurance providers, or underbilling, thereby harming the healthcare provider’s revenue and potentially its reputation. In this context, the system owner could be held liable for negligence for not ensuring the system's accuracy and reliability, directly impacting financial and operational efficiency.
In this scenario, the system owner has a duty of care to ensure that the deterministic rule-based systems it uses is properly maintained, regularly updated, and accurately programmed to reflect current medical billing codes and practices. A breach of this duty occurs if they system owner neglects these responsibilities, leading to a system that generates incorrect billing information. If such inaccuracies result in overcharging patients, underbilling insurance providers, or other financial discrepancies, the causal link between the breach of duty and the resulting damages becomes obvious, fulfilling the causation and damages elements required for a negligence claim.
Additionally, deterministic rule-based systems do not adapt or learn from new data, which means any latent errors in their initial programming or rule sets can persist and propagate over time unless identified and corrected by human operators. The responsibility thus falls on the hospital or healthcare organization to regularly review, update, and verify the accuracy of these systems to prevent errors that could lead to patient harm or financial loss.
The Challenge with Adaptive Learning and AGI Systems
The introduction of artificial intelligence in all its variants brings profound implications for civil legal obligations, especially within the scope of tort law, demanding a new approach to liability considerations, one as innovative as the technology itself. Traditionally, tort law has been based on human actions and ethical responsibility, evident in the concept of the "reasonable person" standard central to negligence law. This principle also extends to strict liability cases, which typically apply to individuals engaging in deliberate activities or failing to prevent a known, preventable situation, where questions of ethical responsibility still emerge.
In scenarios involving traditional machinery, the legal focus is straightforward: determining whether the operators or those in control are personally liable or, in product liability cases, whether the machinery in use was defective. However, the advent of AI complicates this legal regime significantly. As discussed, AI-driven technologies are often designed to function autonomously, without direct human oversight, making their operations opaque even at a basic level. This complexity challenges the traditional negligence framework, which absolves liability if no human could have reasonably foreseen a malfunction, resulting in potentially unfair outcomes. Victims of AI-related damages might find themselves without compensation unless they can appeal to non-fault-based liability theories, such as product liability.
Moreover, the current legal framework creates a paradox where an error causing harm may lead to liability if made by a human but not if made by an AI system, even when the consequences are identical. This discrepancy highlights a critical gap in how the law addresses AI, suggesting a need for reform to ensure fairness and accountability in the age of intelligent machines.
Bridging AI and Human Fault
In the current legal framework, negligence involves a human's action or inaction that fails to meet a specific standard, with liability extending to employers for their employees' faults. However, attributing fault to a machine demands proof of human oversight or error in its programming. This distinction becomes increasingly complex as AI begins to make decisions traditionally made by humans. The principle of compensating victims fairly raises questions about the fairness of dismissing claims due to AI errors while accepting similar claims against human errors. This inconsistency suggests the need for a legal mechanism to attribute liability to AI.
Recent discussions, such as those by the European Parliament regarding legal personality for AI, offer complex solutions that may not address the core issue: the necessity of connecting liability with a human or legal entity capable of compensation. Thus, a practical approach focuses on the outcome of AI decisions, suggesting that if an AI's decision would be deemed negligent if made by a human, then the entity responsible for the AI should bear automatic liability. This approach simplifies the legal response to AI, emphasizing the decision's impact over the decision-making process and introduces the concept of identifying the “person responsible” for the AI's actions, a matter that directly ties to the issue agency.
Agency and Assigning Responsibility in AI-Driven Systems
To make practical the idea of machines being capable of negligence and the proposal that there should be liability for AI-caused harm without direct fault, a legal framework for assigning responsibility is necessary. Since a machine lacks the capacity to own assets for compensating damages or to secure insurance, liability cannot rest with the AI itself despite some courts speculating on the idea. The solution lies in legislating responsibility, aiming to align the costs with those who benefit from AI's use. This approach suggests identifying entities that utilize AI for profit or significant non-commercial activities, excluding private and personal applications.
Two main groups should bear this liability: users and suppliers. Users are those incorporating AI into their operations, such an RCM company using AI for denial management or predictive analytics and decision-support. Suppliers include creators and providers of AI technologies, like software developers or IT firms offering AI solutions. It is reasonable to consider multiple entities jointly responsible for AI's application, enabling claimants to pursue claims against any or all involved parties, with provisions for seeking contributions among them for damages. This structure ensures that those benefiting from AI also bear the financial risks associated with its potential failures.
Identifying Defective AI
Establishing liability for AI-induced damages necessitates a clear definition of what constitutes defective AI. Under the Consumer Protection Act of 1987, a product is deemed defective when it fails to meet the general safety or functionality expectations entitled to consumers. This provides a useful framework for application in RCM processes, emphasizing the need for an objective evaluation of performance unrelated to the producer's fault or the foreseeability of harm. Thus, for RCM use cases, courts must evaluate AI's functionality—whether it performs as intended. Moreover, AI's evolving capabilities pose timing issues for determining defectiveness, as AI can adapt and improve over time, potentially leading to new risks not present at the initial creation.
Therefore, a proposed definition for defective AI should consider both unexpected malfunctions and inherent inadequacies in design, defining AI as defective if it fails to execute its expected function properly and, due to this failure, poses a higher likelihood of causing harm. This definition encompasses both AI systems that exhibit unexpected malfunctions and those inadequately designed or applied from the outset. By focusing on the functional expectations and the potential for operational harm, this approach accommodates AI's dynamic nature while ensuring accountability for AI-related damages.
Conclusion
AI-driven technologies will continue to be an increasingly integral to RCM processes. The adoption of such technologies also brings the potential for errors that could result in financial discrepancies or losses. The reliance on civil liability rules as a regulatory measure for these technologies is increasingly subject to questions and criticism, with discussions emerging across various jurisdictions on the need for specific standards to govern AI applications. These standards aim to ensure a system’s reliability and protect the financial interests of healthcare providers and patients alike.
The conversation extends to how a tort regime can adequately address and attribute liability for malfunctions in AI-driven healthcare financial operations, and in particular, RCM applications. A proposed liability framework suggests aligning with traditional laws of obligations, modifying only to address unique challenges posed by AI technologies. This approach advocates for gradual integration, reflecting the incremental development of AI technologies and allowing for future comprehensive reforms as necessary.
Most legal experts focused on AI liability recommend a strict liability framework for addressing financial injuries stemming from AI in medical billing and collections, alongside a fault-based system for issues related to financial mismanagement. Currently, there's no indication that specialized rules are needed for property damage or other indirect losses related to AI usage in healthcare financial management, as AI does not seem to increase risks warranting additional liability protections. This strategy aims to balance innovation with the need to protect the financial processes of healthcare providers and the billing rights of patients, preparing the ground for future adjustments as the application of AI in healthcare revenue cycle management evolves. There does, however, seem to be traction to develop a collective to compensate for damages to which all stakeholders contribute. A mechanism for establishing and administering such a collective has yet to be realized.
As AI continues to redefine the landscape of healthcare revenue cycle management, navigating the complexities of liability and regulation will be paramount in harnessing its full potential while safeguarding against its pitfalls, ensuring that as we move forward, innovation and accountability go hand in hand in shaping a future where technology serves the best interests of all stakeholders.